The data science revolution: How specialised roles are driving business success
Data science is far from being a catch-all topic. In fact, it evolved into a highly specialised field, with roles ranging from data engineers to machine learning experts and beyond.
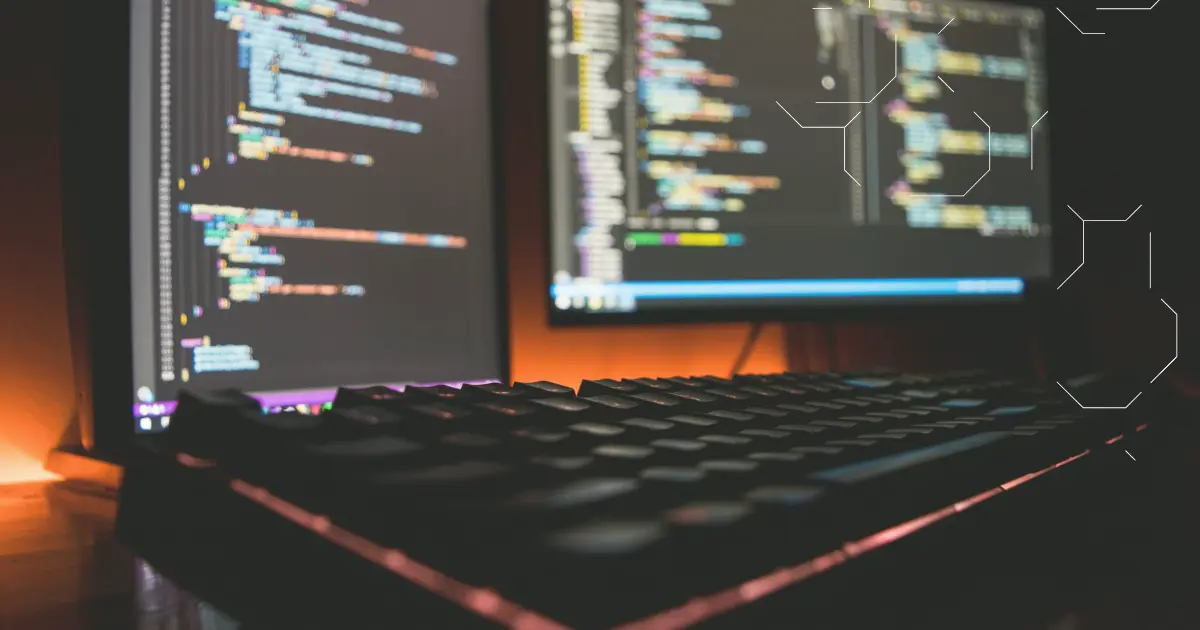
Welcome to the world of data science, where the possibilities are endless and the insights invaluable! But wait, hold on a minute – have you ever heard of the concept of a ‘data science unicorn’?
You know, the elusive individual who can single-handedly manage data, analyse it with machine learning and present it in a compelling visual – all before lunchtime.
Well, it’s time to put that myth to bed. The truth is, data science is far from being a catch-all topic. In fact, it evolved into a highly specialised field, with roles ranging from data engineers to machine learning experts and beyond. And that’s what we’re going to dive into today – so grab a cup of coffee (or tea), get comfortable, and let’s discover the magic of data science specialisation!
The data science unicorn: myth or reality?
With the increasing importance of data in business decision-making, enterprise data teams are now breaking down into specialised roles, each with its own specific responsibilities and skillset.
Let’s start with a common expectation of the fabled data scientist unicorn from only a few years ago:
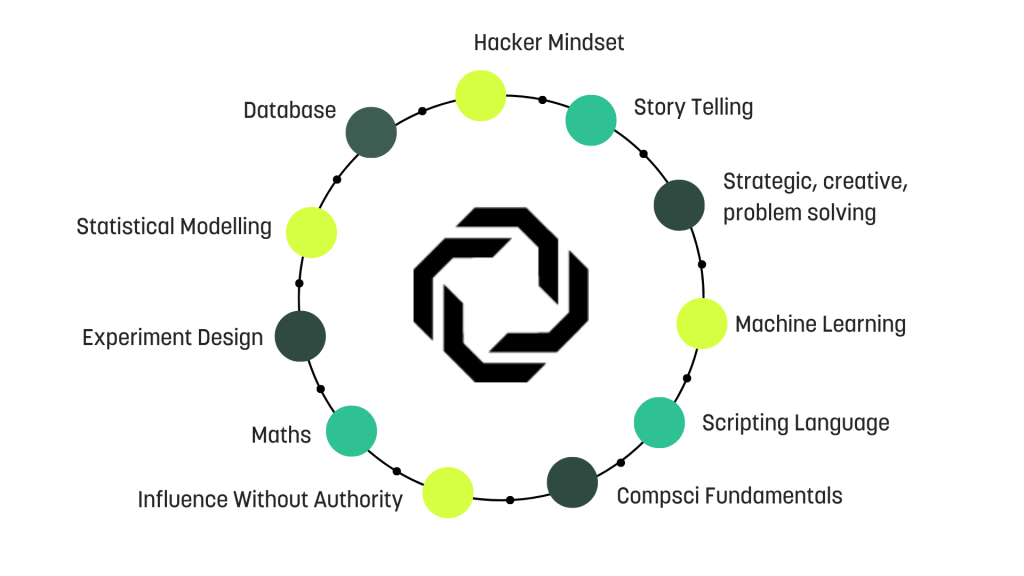
Certainly that’s a lot of capability to pack into an individual.
A “data scientist unicorn,” is an individual who possesses a wide range of skills and abilities in data science. This concept has become a popular topic in the industry. But in reality, it’s highly unlikely to find an individual who is an expert in all aspects of data science.
Also data science is a complex and multidisciplinary field that involves various skills, such as statistics, programming, data visualisation, and machine learning, among others. It is challenging for a single individual to be an expert in all these areas, as it requires years of experience and continuous learning.
Altogether, data scientists are problem solvers. Data science is the practice of working out how to answer plain-language questions about a business with empirical data. What does my audience care the most about? Is my paid advertising delivering an incremental benefit? Who are my most valuable customers? While in theory, this is a simple concept, the techniques and infrastructure needed to realise this ambition are increasingly complex.
In reality, most data scientists, especially in larger FAANG-type organisations, specialise in one or two areas. And they work as part of a larger data science team. This allows organisations to leverage the expertise of multiple individuals and build a stronger and more effective data science capability.
So, let’s explore the specialised roles in data science and what they really mean.
Which are the specialised roles in data science?
These roles differ from each other in terms of their focus, responsibilities, and skill sets. So here’s a deeper overview of each role and when a business might need them the most:
- Data Engineers: Data engineers are responsible for collecting, storing, and organising large data sets. They build and maintain the infrastructure and systems that enable data scientists and analysts to access and analyze data. Businesses might need a data engineer when they are dealing with large and complex data sets that need to be processed and stored efficiently.
- Data Analysts: Data analysts focus on analysing and interpreting data to identify patterns and trends. They work closely with business stakeholders to understand their data needs and help them make data-driven decisions. Businesses might need a data analyst when they need to understand the relationship between different variables and make decisions based on the insights they gain from the data.
- Data Scientists: Data scientists use advanced statistical and machine-learning techniques to build predictive models. They are responsible for identifying the right algorithms and techniques to solve complex business problems. Businesses might need a data scientist when they need to make predictions about future events or behaviours based on past data.
- Machine Learning (ML) Engineers: ML engineers are responsible for designing, building, and deploying machine learning models. They work to understand the business problem, design a solution, and implement it in a scalable way. Machine learning engineers also need to be able to collaborate with data engineers, software engineers, and other stakeholders to integrate the models into the broader technology infrastructure and ensure they deliver value to the business.
- Business Intelligence Analysts: Business intelligence (BI) analysts use data to help organizations make better decisions and improve performance. They work closely with business stakeholders to understand their data needs and develop reports and dashboards that provide them with actionable insights. Businesses might need a BI analyst when they need to monitor key performance metrics, track trends over time, and make data-driven decisions.
How data science roles differ in large and small companies?
The specialisation of roles can differ between large enterprises and smaller agencies based on several factors, including:
Factor | Large Companies | Small Companies |
Size and complexity of data | Typically deal with larger and more complex data sets that require greater levels of regulation and compliance. | Fewer specialised roles and may rely more on generalists to handle their data needs. |
Budget | Often have larger budgets and can afford to invest in dedicated specialists and expensive tech stacks for each role. | May have to rely on a smaller number of generalists who can handle multiple responsibilities. |
Project scope | Larger projects with multiple stakeholders and complex requirements, and may need a specialised team to handle the project. | Smaller projects with fewer stakeholders and simpler requirements, and may only need a generalist or two to handle the project. |
Resource availability | Larger pool of resources and can choose to specialise their data science team based on their specific needs and the type of data they are working with. | Limited resources and may have to rely on generalists to handle the data science needs. |
The level of specialisation in data science roles can differ between large enterprises and smaller agencies. But both can benefit from specialised roles in data science and make the most of their data and drive business success.
Final thoughts
As we celebrate International Day of Women & Girls in Science this week, it’s worth highlighting the importance of diversity in data science.
By breaking down the ‘data science unicorn’ myth and embracing specialised roles, we open the door to new perspectives and solutions. A diverse data science team can bring a range of experiences and expertise, which can lead to better insights, decision-making. And ultimately, a stronger impact on the business.
So, let’s celebrate the women who are shaping the future of data science and the impact they’re making, and encourage organisations to build diverse and specialised data science teams.
We take pride in our partnership with DesignRush, highlighting our dedication to providing exceptional data solutions. To learn more about our extensive expertise and the range of data services we offer, please visit our Data Analytics DesignRush profile.
If you have any questions, get in touch with our data science team.
Considering sharing with others