How AI-driven predictive analytics will future-proof your business
Explore predictive analytics and its power to transform business strategies and drive success across many industries.
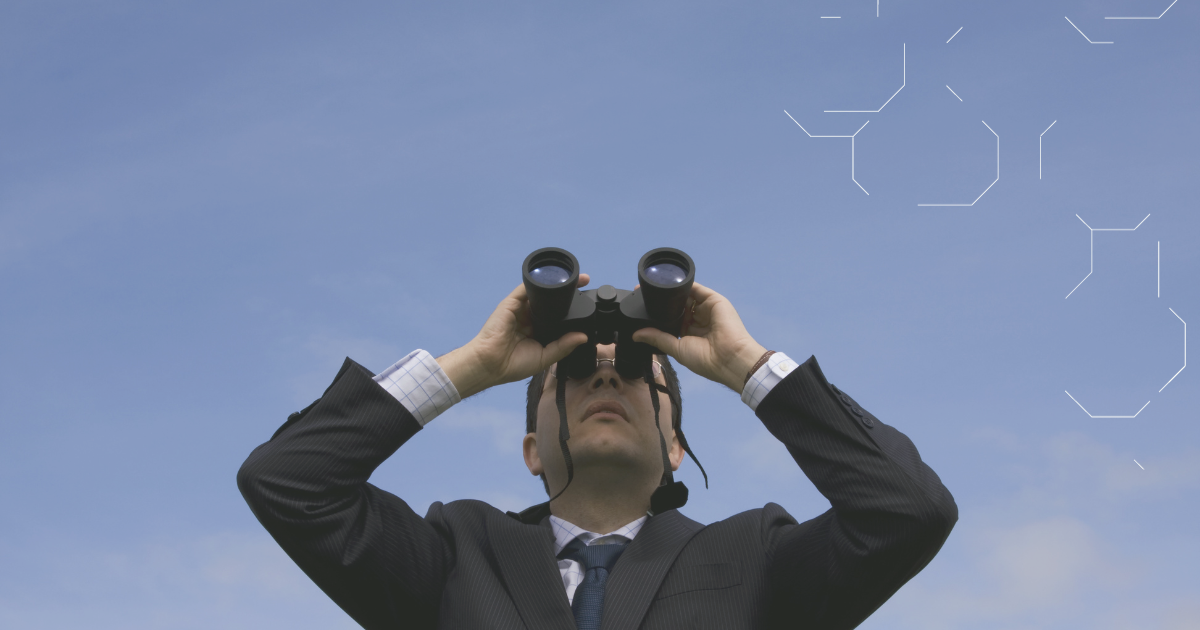
Predicting future events and trends is vital across many industries
With growing volumes of data and easy-to-use software, predictive analytics is more accessible than ever, helping businesses become more proactive and increase their bottom line.
And it appears more often than you might assume — from your weekly weather forecast to algorithm-enabled medical advancements.
Read our article and get started on the path to data-informed strategy and decision-making.
What is predictive analytics?
Predictive analytics (also known as predictive modelling or predictive intelligence) is a subset of data science used to forecast future events. It involves gathering past and current data, a proper study of this data, and then applying statistical or machine learning tools to this data to predict the future needs of any business.
Predictive models have a wide range of uses, including weather forecasts, creating video games, translating voice to text, customer service, and investment portfolio strategies. All of these applications leverage descriptive statistical models of existing data to make predictions about future data.
It allows businesses and investors to adjust where they use their resources to take advantage of possible upcoming events.
Why is it important for businesses?
Rene Davis, Head of Data at Braidr notes: “Predictive analytics is an invaluable tool we use to transform raw data into actionable strategy. Not only can we better understand historic and present behaviour but can be smarter in understanding future trends and customer preferences allowing us to stay ahead of the competition.”
- Strategic insight – it enables businesses to identify which steps through the entire on- and offline customer journey increases the likelihood of a customer converting. By understanding customer behaviour, businesses can make strategic decisions that directly address their target audience’s needs and preferences.
- Improved ROI – it helps businesses allocate marketing budgets according to their most valuable streams. They can pinpoint their most profitable channels, directing funds towards campaigns that yield the highest returns, ensuring better use of resources and increased revenue.
- Leveraged first-party data – Data-driven attribution (DDA) algorithms blend in businesses’ first-party data to get more accurate insights and reporting, leading to better performance.
- Incremental impact – it allows businesses to gauge the indirect effects of their marketing channels. This means they can quantify the ‘halo effect’—or the broader influence—that each channel has. By understanding the incremental impact of their efforts, businesses can better appreciate the full value and reach of their marketing strategies.
3 main techniques in predictive analytics
As more data becomes accessible and powerful, predictive analytics is reaching new levels of precision. Here are three primary methods used in this domain:
- Decision trees
This technique segments data into smaller, uniform groups, and then builds predictive models based on these subsets. Just as the name implies, it looks like a tree with individual branches and leaves.
It can manage both straightforward and intricate relationships. However, its vulnerability to overfitting—where the model might not generalise beyond the training data—demands careful handling, especially with expansive data.
- Neural networks
This model was developed as a form of predictive analytics by imitating the way the human brain works. It’s a self-learning model, widely used for tasks like image and pattern recognition.
You can use it if you have several hurdles that you need to overcome like when you have too much data on hand, or when you don’t have the formula you need to help you find a relationship between the inputs and outputs in your dataset.
- Regression
According to Investopedia, this is the model that is used the most in statistical analysis. It enhances decision processes by pinpointing relationships between a target variable and its influencing factors.
It aims to deduce the best-fit equation explaining these relationships, facilitating future predictions. It’s also valuable for gauging the influence of different factors on the target variable.
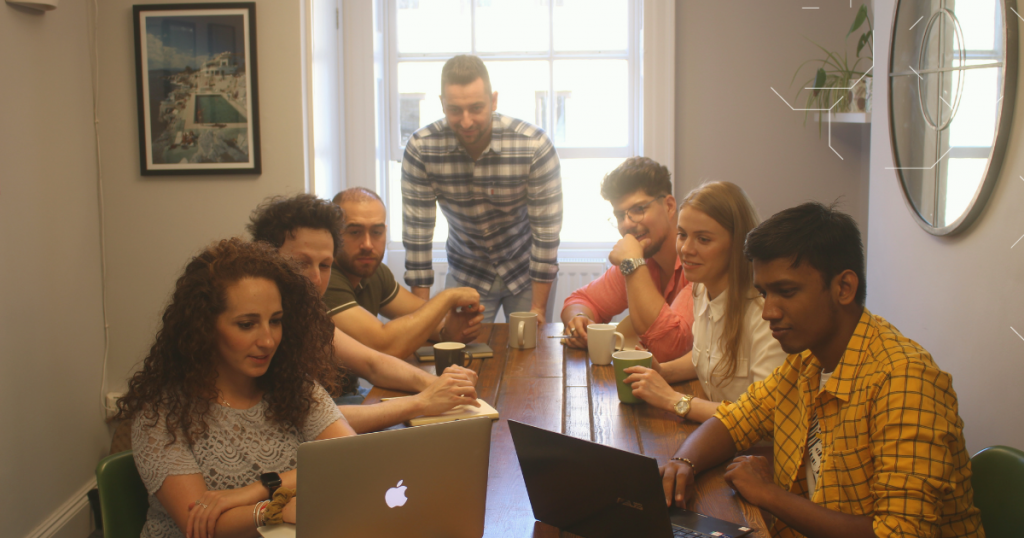
3 examples of how Braidr used predictive analytics
- Generating ad revenue for a large e-commerce retailer
Our client had a vast, rapidly evolving catalogue. Google Ads automated bidding technology struggled to keep up, taking too long to gather enough data to decide how to push every new product, creating stock-keeping units (SKUs) that were effectively dormant. With shoppers never being shown these products, revenue during their key initial release period was much lower than it could have been and often missed out on entirely.
Our team leveraged cloud-based machine learning tech to engineer a deep neural network powerful enough to predict how much Gross Merchandise Value (GMV) a product could generate in a given 30-day period, accurate to within £100.
- Unlocking the power of digital channels for a large catalogue retailer
Our client’s traditionally offline customer base underwent a significant shift to online channels during the pandemic. This transition challenged their long-standing business model, forcing them to reevaluate their strategy. And the increasing costs associated with paper and printing pushed the company to consider reducing its reliance on physical catalogues.
To address these challenges, they needed to gain insights into how their digital marketing channels impacted their revenue, understand their customers’ journeys, and predict their behaviour. We applied data-driven attribution to determine the value of each marketing channel and used marketing mixed modelling to predict their revenue based on their marketing spend.
- Reshaping the customer acquisition strategy for a prestigious hotel chain
Our client wanted to grow internationally, shift focus from brand-specific terms to more general ones, and be acknowledged for innovation in their field.
After the preprocessing part, which is a sequence of steps that prepares the data for ingestion, we helped them by using a well-known unsupervised learning algorithm, clustering. Based on historical data, we identified customer segments likely to reserve accommodations at the hotel. An instruction-based Large Language Model (LLM) was used to translate the customer segments into actionable plans for the paid team.
Final thoughts
The aim of predictive analytics is to make predictions about future events, and then use those predictions to improve decision-making.
To stay competitive, today’s data-driven marketers must leverage innovations including predictive analytics through unified marketing measurement, AI and machine learning.
If you want to put insight behind every marketing decision, we are ready to help. Get in touch with our team today.
Looking for more?_
Related articles
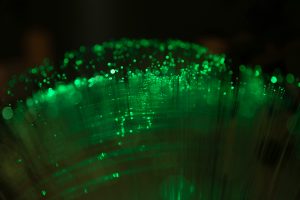
AI-driven predictive analytics: how to turn data into actionable insights
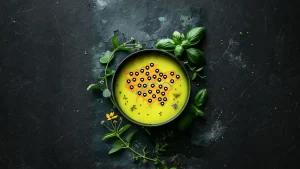